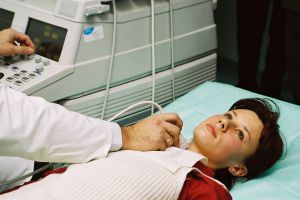
The aim of comparative effectiveness research (CER) is to improve the quality, effectiveness, and efficiency of health care and to help patients, health care professionals, and purchasers make informed decisions. CER is moving forward, with recently defined priorities and a newly funded Patient-Centered Outcomes Research Institute, which we hope will survive congressional cost cutting. To achieve its goals, CER must address the population that consumes the most health care: patients with multiple chronic conditions, especially those with combinations of behavioral and physical conditions such as dementia, mental illness, end-stage renal disease, and heart failure. Such patients account for more than 80% of Medicare costs and are overrepresented in Medicaid and private insurance plans. Ironically, this is also the least studied population. CER provides an opportunity to correct this disparity, but doing so will not be easy.
CER is meant to include representative populations and health care providers, to examine treatment effects within various subpopulations, and to compare interventions head to head. Heterogeneity among patients with multiple chronic conditions complicates each of these features. But this complexity argues for adapting research designs to the important study questions, not ignoring health care’s largest consumers. To accurately inform decision making for patients with multiple chronic conditions, CER must include large, diverse populations representative of those cared for in clinical practice, monitor harms as well as benefits, examine homogeneous subpopulations defined according to risk level, focus on broader health outcomes than clinical research generally considers, and compare interventions that have benefits for multiple health conditions or for overall health (see Steps for Enhancing CER’s Applicability to Patients with Multiple Chronic Conditions).
The almost infinite combinations of diseases, treatments, and other factors affecting health outcomes or treatment responses will make identifying representative study populations a daunting task. The requisite sample sizes and long-term follow-up all but preclude conducting randomized, controlled trials for multiple chronic conditions. Observational research can better accommodate the large, heterogeneous populations needed to examine treatment effects and outcomes under real-world conditions over long periods. However, confounding and bias limit observational studies’ capacity to distinguish treatment effects from the effects of patient-related, disease-related, and provider-related factors. Indication bias is one example (see box); for instance, patients with severe heart failure are likely to have bad outcomes regardless of what treatments they receive. They may also be more likely than patients with less-severe heart failure to receive angiotensin-converting–enzyme inhibitors and less likely to receive beta-blockers — practice differences that make it difficult to disentangle the effect of disease severity from the comparative effectiveness of these two classes of medications. To isolate treatment effects, researchers must consider myriad personal and provider characteristics that are associated with the likelihood of either receiving the study treatments or achieving the target health outcomes.
The heterogeneity of treatment effects will further complicate CER. Although studies typically report average effects, most participants experience more or less benefit and harm than average. Such heterogeneity results from variability in patients’ initial level of risk for a given outcome, in their responsiveness to treatment, and in their vulnerability to adverse effects — issues with particular relevance to patients receiving treatment for multiple coexisting conditions. Treatments must be compared within homogeneous risk strata, defined according to characteristics that affect both benefit and harm from those treatments. If data on these characteristics are available, then relatively homogeneous subgroups can be created for assessing stratum-specific benefits and harms.
The benefits and harms of any treatment may also change over time, as people age and accrue additional conditions and treatments. Current treatment studies rarely last more than 5 years. Changing responses to treatment must be incorporated into CER, necessitating longitudinal studies.
Fortunately, large, representative, and heterogeneous cohorts with well-documented characteristics are or will soon be available for longitudinal observational studies. The Medicare Current Beneficiary Survey (MCBS) and the HMO Collaboratory from the National Institutes of Health (NIH) are examples of relevant sources. In theory, electronic health records (EHRs) would be the ideal source of participants and data, allowing for real-time comparisons of treatment in real patients. To isolate treatment effects, however, EHRs will need to contain more comprehensive, reliable data on health, function, and other variables than they currently do.
Perhaps the most fundamental question is how to define benefit or harm when multiple conditions coexist and multiple treatments are being compared. Primary outcomes tend to be disease-specific — for example, stroke prevention or exacerbation of chronic obstructive pulmonary disease. Such outcomes work well in efficacy studies, which reveal a therapy’s effect on a specific outcome under ideal circumstances in a homogeneous population. They make less sense, however, for comparing treatments in patients with multiple chronic conditions. Treatments that are effective for one disease may exacerbate other diseases or adversely affect overall health. The likelihood of such mixed benefits and harms increases as the number of coexisting conditions mounts. Aggressive antihypertensive treatment, for example, may benefit relatively healthy hypertensive people at any age, but what about a 75-year-old who is depressed, cognitively impaired, and taking 10 other medications? Disease-specific benefits may not be the highest priority for people who are simultaneously at risk for multiple important adverse outcomes.
CER will probably accelerate the movement toward outcome-driven decision making, reimbursement, and quality assessment. As this shift occurs, we must move toward a focus on cross-disease, “universal†outcomes in research and clinical care. Universal health outcomes — outcomes on which all diseases exert an effect — are the consequences that matter to patients.3 Focusing on them would ensure that both harms and benefits of treatments are compared. Examples include symptom burden (e.g., dyspnea, pain, fatigue), function (physical, cognitive, psychological, social), and health-related quality of life. These factors can be assessed by means of patient-reported outcomes or through observed performance or professional evaluation. Because older adults may value independence over longevity, it makes sense to assess active life expectancy, measured over time to capture periods of disability and recovery.
There is no consensus set of universal health outcomes, although health services researchers have been using some key measures for decades. Governmental data sources such as the MCBS, the Long-Term Care Minimum Data Set, and the Outcome and Assessment Information Set for home care also include data on relevant universal health outcomes. Furthermore, federal agencies, including Veterans Affairs, the Center for Medicare and Medicaid Services, the Food and Drug Administration, and the NIH are working on patient-reported outcomes. If such efforts were coordinated, a set of standardized, reliable, and valid cross-disease, universal outcomes could emerge. These outcomes should be incorporated into EHRs, to be used in clinical practice and quality-improvement efforts as well as CER.
Head-to-head comparison is the third key feature of CER. To reduce the complexity of treatments, interventions such as exercise that affect multiple conditions simultaneously should be a high priority. Comparisons might focus on disease-specific versus cross-condition treatments. Studies should include assessment of the burden of treatments for patients and families. Another CER priority should be the examination of treatments for common pairs of diseases in which treatment of one may exacerbate the other. For example, when hypertension and osteoporosis coexist, what treatment best minimizes the risk of adverse cardiovascular outcomes without increasing the risk of falls and fractures? Models of care should also be compared — for example, usual care versus either the medical home or care coordination by nurses during care transitions.
Researchers have largely shied away from the complexity of multiple chronic conditions — avoidance that results in expensive, potentially harmful care of unclear benefit. We cannot improve health care’s quality, effectiveness, and efficiency without addressing its greatest consumers. Development and testing of innovative approaches to care for patients with multiple chronic conditions could prove the most lasting legacy of CER.
Steps for Enhancing CER’s Applicability to Patients with Multiple Chronic Conditions.
Include heterogeneous populations with multiple chronic conditions in sufficient numbers to measure benefits and harms of interventions.
Develop and implement risk-stratification models and report harms and benefits according to risk strata.
Examine universal health outcomes that are relevant across diseases (e.g., function, symptom burden, activity, survival, active life expectancy).
Account for health transitions over time.
Employ analytical methods that account for biases such as confounding by indication (i.e., the indication for treatment is related to the risk of the outcome; those with greater disease severity are more likely to receive a treatment and more likely to have bad outcomes regardless of treatment).
Evaluate longer-term changes in benefits and harms of treatments as patients age and acquire additional conditions. Compare usual care or disease-guideline–driven care with
• Single interventions that affect multiple conditions.
• Innovative models of care.
Evaluate disease pairs, especially those with potential for therapeutic competition (i.e., treatment of one disease may exacerbate a coexisting disease).
Written by Mary E. Tinetti, M.D., and Stephanie A. Studenski, M.D. Dr. Tinetti is a member of the Methodology Committee of the
Patient-Centered Outcomes Research Institute (PCORI), Washington, DC.
The views expressed in this article are those of the authors and not necessarily those of PCORI. This article was originally published here.